Időjárás - Quarterly Journal of the Hungarian Meteorological Service (OMSZ)
Vol. 115, No. 1-2 * Pages 1–122 * January - June 2011
![]() |
Guest Editor: Sándor Szalai |

Editorial
Sándor Szalai
idojaras.2011.1-2.1
Sándor Szalai
idojaras.2011.1-2.1
Mathematical, methodological questions concerning the spatial interpolation of climate elements
Tamás Szentimrey, Zita Bihari, Mónika Lakatos, and Sándor Szalai
idojaras.2011.1-2.2 (p. 1–)
Tamás Szentimrey, Zita Bihari, Mónika Lakatos, and Sándor Szalai
idojaras.2011.1-2.2 (p. 1–)
Aspects regarding the uncertainty of spatial statistical models of climate parameters
Patriche Cristian Valeriu
idojaras.2011.1-2.3 (p. 13–)
Patriche Cristian Valeriu
idojaras.2011.1-2.3 (p. 13–)
Operational maps of monthly mean temperature for WMO Region VI (Europe and Middle East)
Florian Hogewind and Peter Bissolli
idojaras.2011.1-2.4 (p. 31–)
Florian Hogewind and Peter Bissolli
idojaras.2011.1-2.4 (p. 31–)
Time variation of the effect of geographical factors on spatial distribution of summer precipitation over the Czech Republic
Jacques Célestin Moliba Bankanza
idojaras.2011.1-2.5 (p. 51–)
Jacques Célestin Moliba Bankanza
idojaras.2011.1-2.5 (p. 51–)
Geostatistical modeling of high resolution climate change scenario data
Ladislav Vizi, Tomáš Hlásny, Aleš Farda, Petr Štepánek, Petr Skalák, and Zuzana Sitková
idojaras.2011.1-2.6 (p. 71–)
Ladislav Vizi, Tomáš Hlásny, Aleš Farda, Petr Štepánek, Petr Skalák, and Zuzana Sitková
idojaras.2011.1-2.6 (p. 71–)
Interpolation techniques used for data quality control and calculation of technical series: an example of a Central European daily time series
Petr Štěpánek, Pavel Zahradníček, and Radan Huth
idojaras.2011.1-2.7 (p. 87–)
Petr Štěpánek, Pavel Zahradníček, and Radan Huth
idojaras.2011.1-2.7 (p. 87–)
Application of gridded daily data series for calculation of extreme temperature and precipitation indices in Hungary
Mónika Lakatos, Tamás Szentimrey, and Zita Bihari
idojaras.2011.1-2.8 (p. 99–)
Mónika Lakatos, Tamás Szentimrey, and Zita Bihari
idojaras.2011.1-2.8 (p. 99–)
Spatial differentiation of the climatic water balance in Poland
Agnieszka Wypych and Zbigniew Ustrnul
idojaras.2011.1-2.9 (p. 111–)
Agnieszka Wypych and Zbigniew Ustrnul
idojaras.2011.1-2.9 (p. 111–)
News - Farewell to the Executive Editor, Margit Antal
Ernő Mészáros
idojaras.2011.1-2.10
Ernő Mészáros
idojaras.2011.1-2.10
IDŐJÁRÁS - Quarterly Journal
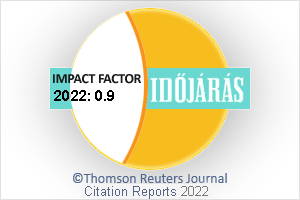
Az IDŐJÁRÁS a HungaroMet Nonprofit Zrt. negyedévenként megjelenő angol nyelvű folyóirata
Megrendelhető a journal.idojaras@met.hu címen.
A szerzőknek szánt útmutató itt olvasható.
Megrendelhető a journal.idojaras@met.hu címen.
A szerzőknek szánt útmutató itt olvasható.