IDŐJÁRÁS - angol nyelvű folyóirat
Vol. 128, No. 2 * Pages 143–286 * April - June 2024

Spatiotemporal imputation of missing rainfall values to establish climate normals
Brian O’Sullivan and Gabrielle Kelly
DOI:10.28974/idojaras.2024.2.6 (pp. 237–249)
Brian O’Sullivan and Gabrielle Kelly
DOI:10.28974/idojaras.2024.2.6 (pp. 237–249)
IDŐJÁRÁS folyóirat
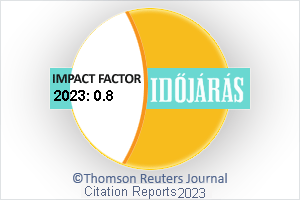
Az IDŐJÁRÁS a HungaroMet Nonprofit Zrt. negyedévenként megjelenő angol nyelvű folyóirata
Megrendelhető a journal.idojaras@met.hu címen.
A szerzőknek szánt útmutató itt olvasható.
Megrendelhető a journal.idojaras@met.hu címen.
A szerzőknek szánt útmutató itt olvasható.