IDŐJÁRÁS - angol nyelvű folyóirat
Vol. 125, No. 4 * Pages 521–692 * October - December 2021
![]() |
Special issue: 30-year anniversary of ALADIN cooperation |

Calibration of wind speed ensemble forecasts for power generation
Sándor Baran and Ágnes Baran
DOI:10.28974/idojaras.2021.4.4 (pp. 609–624)
Sándor Baran and Ágnes Baran
DOI:10.28974/idojaras.2021.4.4 (pp. 609–624)
IDŐJÁRÁS folyóirat
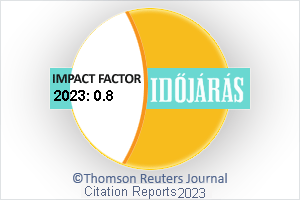
Az IDŐJÁRÁS a HungaroMet Nonprofit Zrt. negyedévenként megjelenő angol nyelvű folyóirata
Megrendelhető a journal.idojaras@met.hu címen.
A szerzőknek szánt útmutató itt olvasható.
Megrendelhető a journal.idojaras@met.hu címen.
A szerzőknek szánt útmutató itt olvasható.